Salespeople are in one of the toughest professions with high workloads. They can spend up to 33% of their day writing emails or on calls, 12% of the day is occupied in internal meetings, 17% on entering data to create reports and that leaves them only 17% of the time to prospect new leads. One of the findings published by Salesforce in their State of Sales report says that high-performing sales teams have been found to use sales intelligence tools for smart sales and reducing repetitive manual tasks.
How machine learning is shaping sales and surplus
Sales is a business unit that is required to ensure that selling is carried out efficiently and intelligently so as to deliver the most effective return on the company’s products and services. It is one of the foremost business areas that have and will continue to benefit the most from AI tools.
Companies that used AI and machine learning in their sales strategy saw their leads increase by more than 50%, call time decrease by 60-70%, and cost reduction by 40-60%.
The sales function relies significantly on predictions and insights. Not surprising then that companies that used AI and machine learning in their sales strategy saw their leads increase by more than 50%, call time decrease by 60-70%, and cost reduction by 40-60%. Read on to find out how this was made possible.
1. Sales forecasting
Sales intelligence tools leverage machine learning uses to accurately predict sales. ML models learn patterns from the data to generate predictions. The prediction algorithm can be run on a cloud machine learning environment or a virtual machine. Predictions are written directly to a database and then distributed to business users through interactive dashboards. One source of truth for the entire company makes sales and reporting more transparent.
Sales forecasting using ML is time-series regression dependant. Time-series regressions are tasks concerned with the estimation of a continuous quantity (sales) with an additional time dimension and are of two types. The choice of model is dictated by the business problem at hand, data availability and a rigorous model testing process.
- Auto-regressive models likeauto-regressive integrated moving average (Arima), seasonal auto-regressive integrated moving average (Sarimax), and exponential smoothing predict future sales exclusively based on values of past sales and generate predictions by finding trends and seasonality patterns.
- Multivariate models include linear regressions, neural networks, decision tree-based methods and support vector machines. Here models are based on a variety of inputs, including past sales, holiday calendars, or even economic indicators.
Also Read: 5 Ways Automation Is Improving Customer Experience
2. Lead scoring
If your sales personnel are focusing on prospects that may not be likely to actually become your customers, then a lot of effort is being wasted in a lost cause. Nowadays, ML-based CRM applications help your sales force to identify the best potential customer prospects by using a well-defined set of attributes and characteristics to predict likely conversions. After all, with so much data available about potential customers, this process of scoring leads can be very efficient for converting leads into actual sales.
Businesses that use AI-enabled CRM software can analyze every aspect of a customer’s relationship to build detailed customer profiles and identify crucial moments in the sales process.
3. Data analysis and pricing
Using data analysis, you can predict the customer churn ratio, devise efficient pricing models, rework your business models or products and services, so that they are better tailored to meet market demand. Given that machine learning can analyze enormous quantities of data in no time, such data can be used for generating insights that will aid you in taking better business decisions.
Pricing is of course usually the final straw that makes or breaks a sale. Traditionally, sales reps, fish in a sea of data to figure out which price is most likely to be the bait the customer snaps at. They rely heavily on intuition and gut instincts, which doesn’t always work well.
Now, those huge amounts of historical data can be fed into a price automation solution, powered by machine learning, capable of automatically pricing products at scale. The model uses historical data such as past and current pricing data, discounts, purchasing history as well as unstructured data such as images and text. It learns the pricing rules with no explicit coding to calculate the optimal price for each customer giving your sales team the edge for closing the deal.
For instance, machine learning is a part of every aspect of Amazon’s business operations, from moderating spam and content discovery to monetizing advertising and reducing churn of customers by targeting offers and products of interest to them. A level of personalization that has been followed by other sales teams.
Also Read: Robotic Process Automation vs Traditional Automation
4. Improving productivity by automating repetitive tasks
AI and machine learning have been able to automate repetitive and time-consuming tasks and go a long way towards reducing monotony and making sales teams more efficient. Machine learning can take over tasks such as sending automatic messages, assigning a call, scheduling a meeting, updating CRM profiles – all mundane yet necessary tasks for salespeople. Sales teams freed from carrying out these tasks can focus on sales pitches, better networking, and sales analytics.
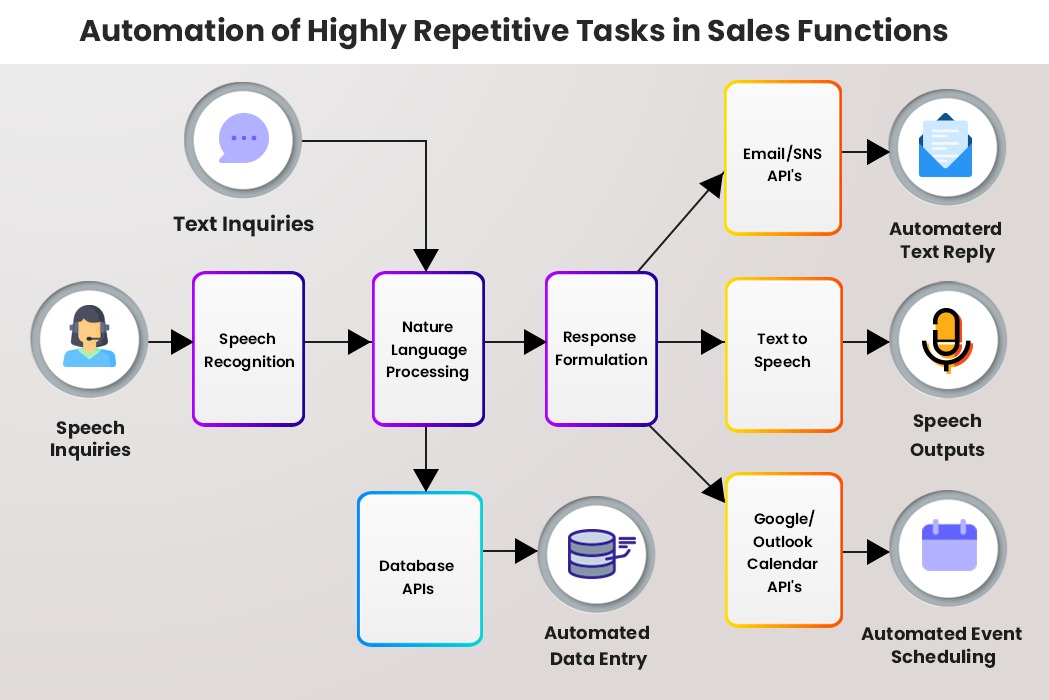
5. Innovative use of consumer data
Businesses are interested in utilizing their large volumes of consumer data to improve profits and future growth. Over the past decades, companies have collected billions of data points on their customers including information like shopping habits, demographic identifiers, income, and more.
Now is the time to actually utilize this data using sales analytics software that can collect information and generate useful and actionable predictions.
Netflix is an online streaming platform that uses machine learning to build extensive view profiles that accurately predict which shows and movies users will be interested in. Netflix saved $1 billion due to its ML algorithm from the combined effect of personalization and content recommendations.
6. Increased customer acquisition
One of the mainstays of enhanced revenue generation for businesses in any sector is acquiring new customers. Companies are relying on applying machine learning techniques to their CRM data to discover better selling strategies that could attract new buyers. Sales teams are better able to anticipate market trends, be prepared for customer queries, and have a holistic product or service overview.
For example, the online retail marketplace Etsy uses machine learning to improve its customer experience by creating individualized customer profiles, improving search results and user design. Its innovative use of data analytics is a compelling reason for its annual revenues of $603 million while facing stiff competition from larger retail companies like Amazon and Target.
In addition, advanced analytics with machine learning can be used as sales performance management technology for ensuring accuracy in the automation of commission processing.
The future of machine learning in sales
Machines are continuously learning so we can expect that AI tools will become more and more accurate and efficient with access to more data. The future could also see all repetitive tasks being carried out by AI tools with the human sales force able to concentrate solely on their goal of onboarding new customers.
As technology is increasingly automating the world, we humans tend to feel threatened. Will sales teams be increasingly replaced by AI and machine learning? The human factor in sales is irreplaceable. People are social creatures and we almost always want to have a human interaction before we actually purchase anything, especially big-ticket items. Machine learning takes over the mundane administrative tasks while giving sales teams the invaluable gift of time to do their principal function – selling.