The increasing application of AI and Machine Learning Technologies in American healthcare throws up concerns with Personal Identifiable Information PII. As we continue the quest for innovative healthcare solutions, the protection of sensitive patient information remains paramount. Enter data masking and data anonymization — two pivotal techniques that are reshaping the way we harness the power of artificial intelligence (AI) in healthcare.
In the United States, where healthcare data is vast and diverse, the need to balance cutting-edge medical advancements with strict patient privacy regulations like the Health Insurance Portability and Accountability Act (HIPAA) is of utmost importance. This delicate equilibrium is precisely where data masking and data anonymization shine.
Here are important insights into these specific technologies, understanding how they are revolutionizing healthcare AI solutions while ensuring the security of patient data.
What is Data Masking in Healthcare?
Data masking is a technique that cloaks sensitive information within a dataset to prevent unauthorized access or exposure. When it comes to healthcare, this can encompass patient names, social security numbers, medical records, and other personally identifiable information (PII). The aim is to retain the utility of the data for research, diagnosis, and treatment while safeguarding the individual’s privacy.
The Technology Involved in Data Masking in Healthcare:
- Tokenization: Cutting-edge tokenization algorithms replace specific data elements with tokens or placeholders. For instance, a patient’s name is substituted with a unique identifier while maintaining the data’s structural integrity.
- Format-Preserving Encryption (FPE): FPE technology encrypts data while preserving its format, ensuring that masked information remains compatible with existing systems and processes.
- Data Redaction: Redaction tools allow for the selective removal or blacking out of sensitive information in documents, images, or records.
What is Data Anonymization in Healthcare?
Data anonymization goes a step further than data masking by transforming data in such a way that it becomes exceedingly difficult to trace it back to an individual. In American healthcare, this means ensuring that even with access to extensive medical datasets, patient identities remain concealed while enabling innovative AI-driven healthcare solutions.
The technology Involved in Data Anonymization for healthcare:
- K-Anonymity: K-anonymity algorithms modify data to ensure that at least k individuals in a dataset share the same set of attributes, making it nearly impossible to identify any specific patient.
- Differential Privacy: Leveraging differential privacy techniques, data is intentionally perturbed or noised, protecting individual records while allowing meaningful insights to be extracted.
- Secure Multiparty Computation (SMC): SMC protocols enable multiple parties to jointly compute functions over their inputs without revealing those inputs, making collaborative data analysis highly secure.
What is the difference between Data Masking and Data Anonymization?
Let’s illustrate the difference between Data Masking and Data Anonymization with examples related to healthcare data.
Suppose you have a patient’s name, “John Smith,” in a healthcare dataset. During Data Masking, you would replace the name with special characters, resulting in something like “**** *****.” This obscures the actual name while maintaining the data’s format.
Data Masking is the option used in scenarios where you need to share data with authorized individuals or departments internally e.g., masking credit card numbers. Data masking is often applied when sharing datasets with development and testing teams to ensure that sensitive information remains protected during these stages, without changing the information for analysis.
In the case of Data Anonymization, you would replace “John Smith” with entirely fictitious data that still resembles the original record. For instance, you might replace it with “Michael Johnson” or “Sarah Brown.” This ensures that the data is not linked to any real individual, even though it looks similar to the original information.
Data Anonymization is essential in cases where strict data privacy regulations like GDPR or HIPAA, must be adhered to or when sharing with external research teams. Data anonymization is often the preferred method to avoid legal issues related to data sharing.
These processes, Data Masking, and Data Anonymization are usually carried out as part of the data cleansing and preparation phases in AI development, before datasets are shared with the broader team.
Use Cases of Data masking and Data Anonymization in Healthcare
While AI is increasingly finding new applications in healthcare. Here are a few ways PII data masking and data anonymization methodology is practically applied.
1. AI-Powered Disease Diagnosis
AI-powered disease diagnosis is important as it enhances diagnostic accuracy, efficiency, and speed. It aids healthcare professionals by analyzing patient data, including clinical notes and medical images, to identify patterns and anomalies. Even smaller medical practices are increasingly considering AI-based diagnostic tools as part of their Electronic Health Records (EHR) systems to improve diagnostic capabilities and provide more accurate treatment recommendations.
Data Masking Techniques Used
- Pseudonymization: Replaces patient names with unique, randomly generated codes, ensuring that individuals cannot be identified by their names.
- Generalization: Reduces the granularity of data, such as age, by grouping it into broader categories (e.g., 30-40 years old) to prevent precise identification.
- Tokenization: Replaces specific medical terms or codes (e.g., ICD-10 codes) with tokens, ensuring that the medical condition or treatment remains confidential.
2. Remote Patient Monitoring AI integration
Remote Patient Monitoring (RPM) is vital for improving patient care by enabling real-time health monitoring outside healthcare facilities. AI plays a crucial role in RPM by analyzing continuous patient data, predicting health events, and tailoring care plans. RPM enhances patient engagement, reduces healthcare costs, and ensures timely intervention, especially for chronic conditions. AI-driven insights help healthcare providers deliver proactive and personalized care, making RPM an essential component of modern healthcare.
Data Masking Techniques Used:
- Secure Transmission: Uses encryption protocols like TLS/SSL to secure data in transit between wearable devices and cloud servers.
- Data Truncation: Limits the data transmitted to essential information to minimize the risk of exposure.
3. Population Health Management
Many large health systems and hospitals have dedicated departments or teams focused on population health management. They often serve as the primary entities responsible for managing the health of the communities they serve. These can be health insurance companies, Integrated Delivery Networks (IDNs) which include networks of hospitals, clinics, and other healthcare facilities; and of course public health agencies.
Data Masking Techniques Used:
- Data Aggregation: Aggregates data to higher levels (e.g., zip codes or regions) to reduce the risk of identifying individuals in smaller populations.
- Data De-identification: Removes or masks specific identifiers like names, addresses, and social security numbers.
Data Anonymization Techniques Used:
Differential Privacy: Applies differential privacy to aggregated population health data to ensure individual privacy while enabling meaningful population-level analysis.
4. AI in Radiology and Medical Imaging
AI in radiology and medical imaging is widely used. It assists radiologists in analyzing images, improving efficiency, accuracy, and early disease detection. AI also streamlines workflows and plays a crucial role in telemedicine, research, and development in healthcare. Its adoption is expected to keep growing.
Data Masking Techniques Used:
- Pixelization: Blocks out or pixelates regions of medical images that do not pertain to the diagnosis to obscure sensitive information.
- Metadata Removal: Strips metadata and any patient-identifying information from DICOM (Digital Imaging and Communications in Medicine) files.
Data Anonymization Techniques Used:
- Data Perturbation: Introduces random noise to pixel values in images while maintaining diagnostic quality.
- K-Anonymity: Ensures that each medical image corresponds to at least k individuals, making it difficult to identify specific patients.
K-Anonymity is a privacy-preserving technique used in data anonymization to protect individual identities in datasets. It ensures that each individual’s data is indistinguishable from at least “k” other individuals’ data points, making it challenging to identify specific individuals based on the available data.
Set up a demo with our senior team to understand more about how we ensure the privacy of your data when developing AI solutions for your organization.iTech has a proprietary EHR platform, RehabONE,that is deployed for American healthcare organizations. We are HIPAA and GDPR-compliant. iTech is also ISO27001, ISO27701, and SOC2 certified!
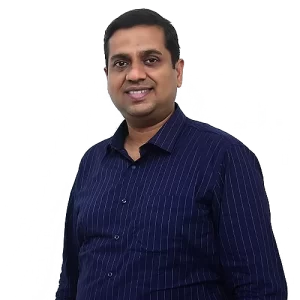
Navin Kumar Parthiban
Navin Kumar Parthiban is a seasoned professional in the field of AI technologies and is a Director at iTech India. With a passion for innovation and a keen understanding of the ever-evolving landscape of artificial intelligence, Navin has played a pivotal role in driving iTech India’s success and technological advancements. Navin regularly shares his insights and knowledge through articles, seminars, and workshops. He believes in the power of AI to revolutionize industries and improve people’s lives, and he is dedicated to staying at the forefront of this rapidly evolving field.