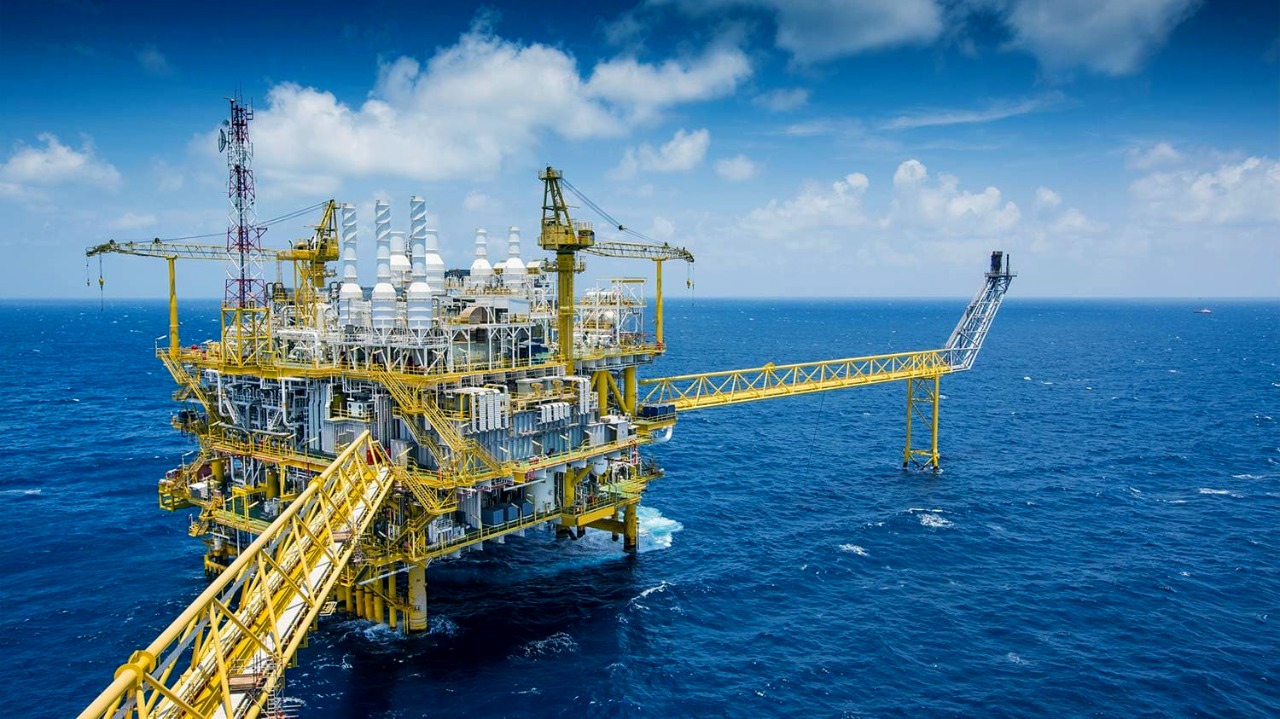
India is the third largest in oil consumption globally and the second largest refiner in South Asia. This makes it imperative that Indian petroleum and alternative energy sectors join other industries that have already seen cost saving through adoption of ML induced efficiencies.
Data, Data everywhere but…
“Data is the new oil. It’s valuable, but if unrefined it cannot really be used.”
The British mathematician, Clive Humby, got it exactly. The petroleum industry has no dearth of data but when it sits in different silos in different formats it becomes the biggest barrier to it being utilized as a single truth.
The challenges in adoption of Machine Learning in the Oil and Gas Industry
1. Closed systems
Much of the data originates from suppliers as well as partner services. These suppliers tend to lock-in their customers by using fully integrated systems that use a proprietary data format that do allow open API access. Sensor based equipment can generate a lot of data but it can often lie unused outside the small window of its localized application. The oil and gas companies are demanding that equipment for future procurement comply with standards-based specification that is secure and interoperable. Big data in oil and gas is the fuel towards numerous applications in upstream, midstream and downstream operations.
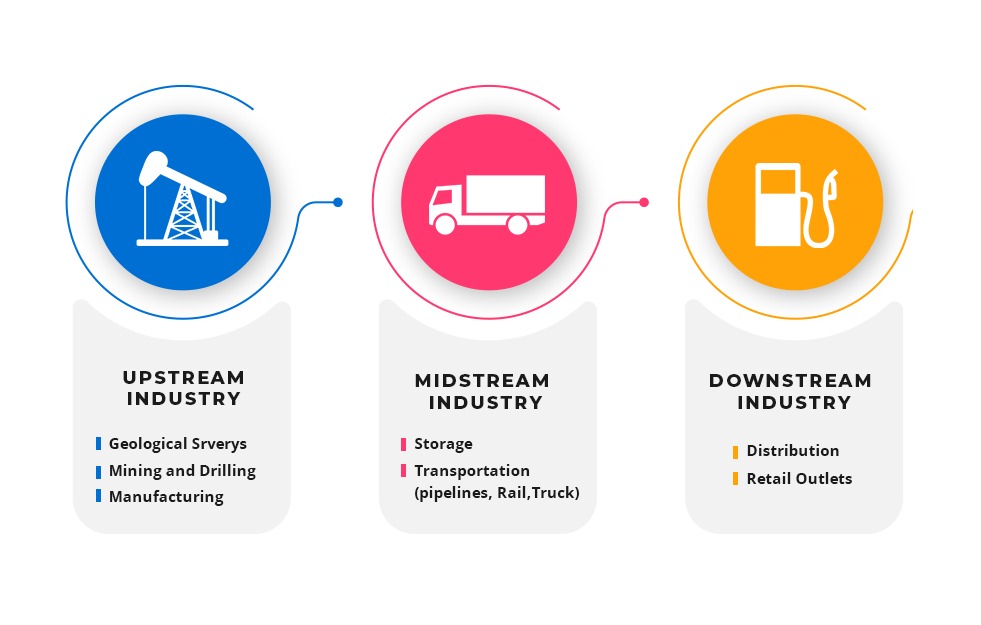
2. Dark data
In simple terms, dark data is any data that has not been tagged and therefore not searchable. It often refers primarily to raw text-based data that has never been analyzed. In the oil and gas sector, like any other industry, almost 83% of information generated is considered to lie in this black hole. This could be seismic data, drilling reports, well data as well as multidisciplinary information on geology, hydraulics; and apparently unrelated information in the digital universe such as news reports and social media posts that could throw light on emerging political information or environmental issues.
Deep semantic technology has been able to make sense of this disparate data, to solve problems before they can occur rather than be reactive. This massive volume of data can be moved into data lakes by tagging the information to make it comprehensible, searchable and usable by different applications. Semantic technology uses natural language processing NLP to add reasoning and contextual knowledge to data, making it suitable for new application in oil and gas analytics.
3. High cost of failure
The most important factor towards profitability is to maintain uptime. Companies are worried that downtime due to technology failures can lead to loss in millions. In fact, a study by Kimberlite has shown that a 1% unexpected downtime can cause an O&G company a loss of $5.037 million each year. This is an important consideration, making the petroleum business take their time to consider all factors before adoption of any new technology.
Also read: The Importance of Data Processing in Machine Learning
Big data needs Data Lakes for optimal outcomes
New data types require a new storage system to handle them and Data Lakes has proven to be the answer. A Data Lake allows storage of data in its original format and can be deployed in the cloud. This translates to the ability to store a massive amount of data be it structured, semi-structured or unstructured data.
The idea behind usage of Data Lakes is that legacy databases are not a good fit for big data. This is not only because of the size of the data but also because much of the data cannot be normalized. A data lake can store raw data in its native format along with relevant metadata. This way, while not all data might get extracted for immediate use, the data remains in a searchable format that allows for its exploitation anytime in the future.
Also read: How Businesses Use Machine Learning to Improve Processes
Data Lakes and PA can help level the playing field
The promising part of new advances in data technology is that smaller companies need not be daunted by the costs involved in adopting Big Data and Machine Learning in oil and gas sector.
Gone are the days when only the giants could afford costly in-house storage systems. Just as personal computers displaced mainframes, so too cloud computing has made it a level playing field in the energy sector. Microsoft Azure, AWS (Amazon Web Services) and Google Cloud have infinite storage and AI and ML to store and consume your data.
You need a technology partner to help you clean and make sense of your data. iTech India is the partner of choice for industries in various sectors, both in India and the USA.