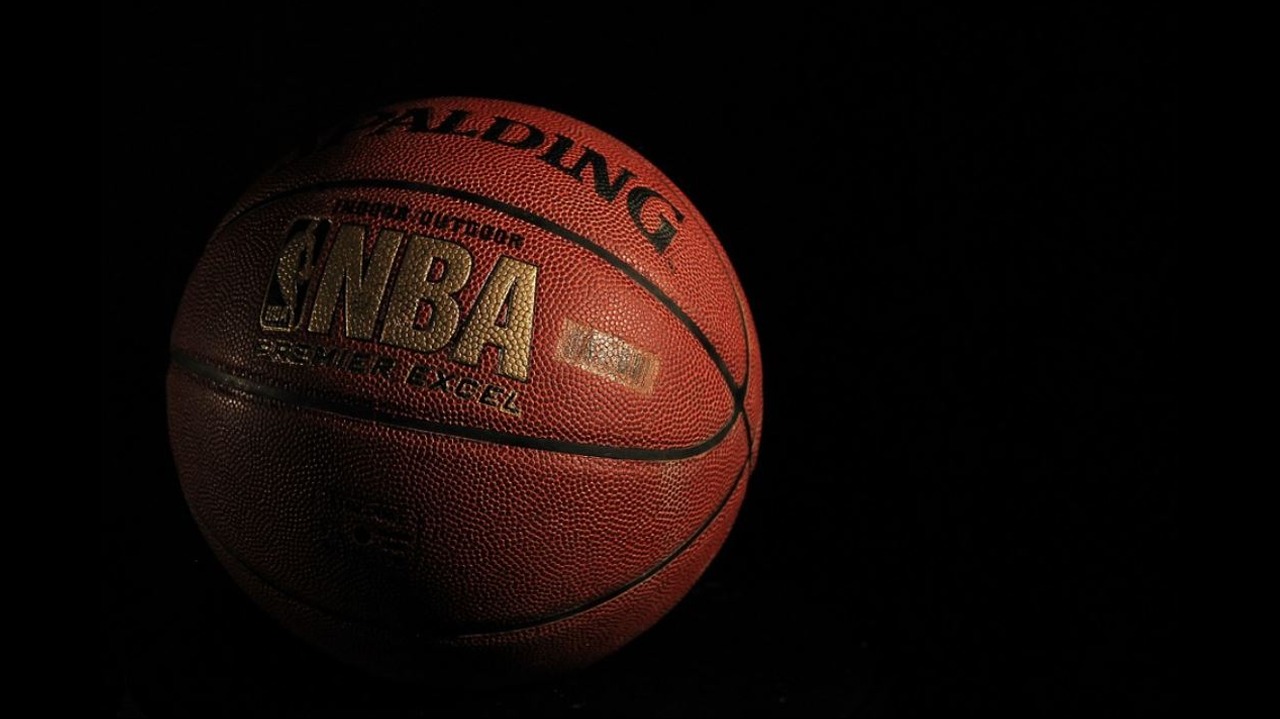
Sports analytics based on deep learning has permeated all sports and even related industries like media. The NBA even has basketball machine learning analytics helping its scouting department to gain as much information as possible on prospects.
It is not just the bigwigs who have the wherewithal to invest in deep learning in sports. Many tech companies have developed basketball machine learning solutions that help sports at different levels. Artificial intelligence in basketball has percolated right down to talent scouting at school level so that colleges and pro teams can access data to scout talent that they might never have been aware of otherwise.
Basketball machine Learning making a play
5 players step on to the court, each of them has a pivotal role to play and each of them have specialised skills. We’ve had all-time greats at these positions
Magic Johnson at 1 position as Points Guard for The Los Angeles Lakers was the guy with excellent court vision and set up the scoring opportunities.
The 2 position or Shooting Guard shoots from all positions and especially from the three-point range. The skills a talent scout is looking for is superior ball handling, jumping skills, excellent defence with a high free throw percentage. 6-time NBA Champion, Michal Jordan anybody? And Kobe Bryant fans will not let us forget him either.
Position 3 or the Small Forwards are the tall dudes and score like the shooting guards. It is a versatile position and the best small forwards are superior scorers around the rim and beyond the three-point line.
The 4 Position (Power Forwards), usually play offense with their back to the baskets and so they are also the best rebounders on the team. Giannis Antetokounmpo of the Milwaukee Bucks is the top ranked NBA power forward this season 2020.
Position 5 (Center) are traditionally the largest and strongest players on the team and are powerful in the middle and excellent shot blockers as well as rebounders. And that is what made Kareem Abdul- Jabbar a Hall of Fame inductee.
In modern basketball these positions have become blurred and NBA players often merge 2 or even 3 roles on court. Machine learning methods have quantified entirely new positions based on statistical contributions that players make on the court. This evolving style of playing has brought artificial intelligence into basketball talent scouting.
Talent scouting should not miss out on talent
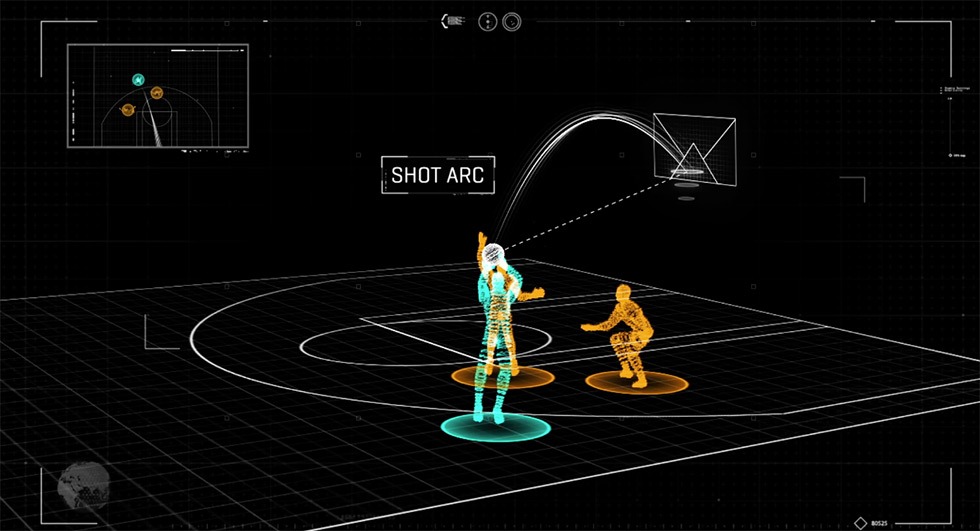
Talent scouts watch players at every level of play, right from middle school and paying the highest attention to high school and college players. Scouts are evaluating players on shot selection and passing opportunities, rebound, dribbling skills and defensive capabilities. If they like what they see over many games then they might make a player an offer. Sounds simple? But many talented players might be missing out on opportunities because they might not be on a scouts’ radar.
There are 341 Division 1 college teams and over 800,000 boys and girls in high school basketball teams in the US. This is why deep learning in sports is starting to muscle its way in to basketball talent scouting at school and college level.
Computer vision and machine learning algorithms analyse players performance statistics and game videos to provide in-depth analytics of many more players than was possible before, pointing scouts to the right direction; often to kids that they might never have seen otherwise.
Also Read: From Data to Decision Making – How Machine Learning is Impacting Sports
Big Data changing the way talent is scouted
Data is easily available and this is why more tech solutions are coming into the sports arena and developed at different price points. For example, game film from NBA, Division 1 college basketball and other leagues are a good source of visual information. Computer vision is the technology that is enabling computers to gather and analyse this visual data. Another source of data is basketball-reference.com where the NBA stats of all players both rookie and pro for over 20 years can be scraped and formatted into data-frames on Python.
Having all of a new player’s game film and running it against standardized patterns can compare their ability with known parameters. For example, to evaluate the shooting ability of a player, coaches and scouts requires a player to make numerous shots. More the shots the better the assessment. However, trained algorithms can now recognize the characteristics of a bucket and can provide an accurate estimation of a player ability based on fewer shots.
Machine learning algorithms in basketball talent scouting is finally doing what humans do very well – spotting patterns – but doing it on hundreds of hours of video footage. The impact of artificial intelligence in Basketball (or any sport) is in automation and removing human bias. Though with stats to back them, decisions will always finally come down to humans.
iTech has worked on developmental projects to track athlete fitness and talent scouting. If your team is interested to develop technology solutions, Talk to us.